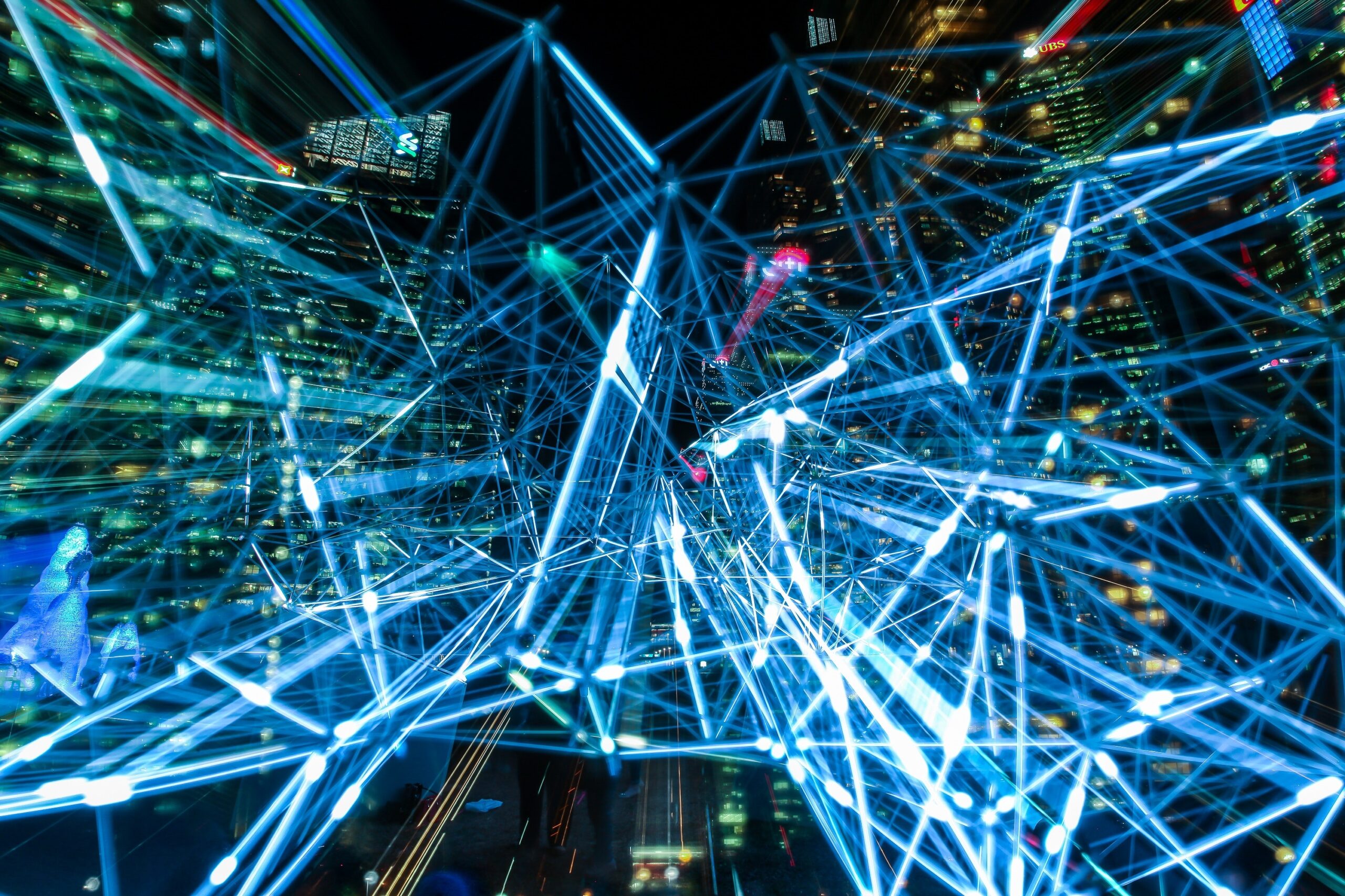
The following editorial will be featured in an upcoming issue of The American Journal of Bioethics
Just last week (October 4, 2022), the U.S. White House released a blueprint for an A.I. Bill of Rights, consisting of “five principles and associated practices to help guide the design, use, and deployment of automated systems to protect the rights of the American public in the age of artificial intelligence.” The white paper states, “Developed through extensive consultation with the American public, these principles are a blueprint for building and deploying automated systems that are aligned with democratic values and protect civil rights, civil liberties, and privacy.” It further articulates that, “this framework provides a national values statement and toolkit that is sector-agnostic to inform building these protections into policy, practice, or the technological design process. Where existing law or policy—such as sector-specific privacy laws and oversight requirements—do not already provide guidance, the Blueprint for an AI Bill of Rights should be used to inform policy decisions”.
I applaud the development of this blueprint, but, after briefly describing each principle, highlight some challenges and questions that bioethicists working on AI and machine learning in health care ought to consider.
Safe and Effective Systems: Protection from Unsafe or Ineffective Systems
“Automated systems should be developed with consultation from diverse communities, stakeholders, and domain experts to identify concerns, risks, and potential impacts of the system. Systems should undergo pre-deployment testing, risk identification and mitigation, and ongoing monitoring that demonstrate they are safe and effective based on their intended use, mitigation of unsafe outcomes including those beyond the intended use, and adherence to
domain-specific standards. Outcomes of these protective measures should include the possibility of not deploying the system or removing a system from use”.
One interesting thing to note about this principle is its framing—on the face of it, it seems to primarily concern protection from something—unsafe or ineffective A (a “negative right”). But there is another way to think about it, and that is, at some point, in some circumstances, patients may have a right to AI systems (a “positive right). We should not lose sight of this. While it is true that AI has the potential to be harmful and unsafe, it also has the potential to be much safer and more effective than human systems and decision-makers (which have their own biases and flaws). I am happy to see this point touched upon via the emphasis on comparing AI outcomes to the outcomes of non-deployment for the sake of a full harm-benefit analysis.
Algorithmic Discrimination: Protection from Discrimination by Algorithms and Algorithms Should be Used and Designed in an Equitable Way
Algorithmic discrimination occurs when “automated systems contribute to unjustified different treatment or impacts disfavoring people based on their race, color, ethnicity, sex (including pregnancy, childbirth, and related medical conditions, gender identity, intersex status, and sexual orientation), religion, age, national origin, disability, veteran status, genetic information, or any other classification protected by law. Depending on the specific circumstances, such algorithmic discrimination may violate legal protections.” The blueprint further explains that,
“This protection should include proactive equity assessments as part of the system design, use of representative data and protection against proxies for demographic features, ensuring accessibility for people with disabilities in design and development, pre-deployment and ongoing disparity testing and mitigation…”.
A key word in the articulation of this principle is “unjustified”—the assertion is that people have a right to protection from “unjustified different treatment” by the algorithm. But what does “unjustified” mean, from an ethical perspective? Imagine that an AI based algorithm predicts how long a patient will live with a left-ventricular assist device (a project my team and I are currently working on). Imagine that this algorithm shows that, on average, patients of a certain race or age range do worse with the device, thereby leading less of these patients to be offered and have access to the device. In doing this, the algorithm contributes to different treatments and impacts, disfavoring those in certain groups. But is this “unjustified” disfavoring or not? This depends largely on your ethical views on justice. On some views it would, on others it would not.
Data Privacy: Protection from Abusive Data Practices Via Built-In Protections and Agency Over How Data Is Used
This principle stresses the importance of consent for data collection and usage, privacy by design defaults where consent is not possible, and freedom from surveillance technologies (e.g., at work/on your work computer).
I agree that this principle is critical and fundamental—the right to privacy is not a new or novel right, but in the context of the vast amounts of data required for the construction and maintenance of AI systems, it cannot be stressed enough. This principle goes beyond privacy in its scope, however, and extends to data ownership. One caveat in this principle is its explicit call for defaults towards privacy, stressing that “design decisions that obfuscate user choice or burden users with defaults that are privacy invasive.” While I agree that in many contexts (e.g., social media use, marketing), such defaults are appropriate, in the medical context, defaults against data sharing may do more harm than good. Data are needed to help improve and maintain the very systems in question—AI and machine learning systems are data hungry. In essence, strong defaults in favor of data privacy and against data sharing could be in tension with the “safe and effective systems” principle/right.
Notice and Explanation: Knowledge That an Automated System is Used and Understanding of Impacts
“Designers, developers, and deployers of automated systems should provide generally accessible plain language documentation including clear descriptions of the overall system functioning and the role automation plays, notice that such systems are in use, the individual or organization responsible for the system, and explanations of outcomes that are clear, timely, and accessible. Such notice should be kept up-to-date, and people impacted by the system should be notified of significant use case or key functionality changes. You should know how and why an outcome impacting you was determined by an automated system, including when the automated system is not the sole input determining the outcome. Automated systems should provide explanations that are technically valid, meaningful and useful to you and to any operators or others who need to understand the system, and calibrated to the level of risk based on the context”.
While I agree in principle with the benefits of transparency and disclosure, in the context of health care AI and machine learning, it is unclear how and why notice and expatiation of use of an AI-based system ought to be achieved with patients. Mere disclosure that an automated system was used as part of care will likely mean little to patients and explanation of its technical details and risks/benefits will likely overwhelm patients, even if done at a big-picture level. In my own research in this area, my team has found that, ultimately, patients trust their physicians and if their physicians are using AI-based systems, they trust them. Moreover, standard practice with non-AI based systems does not require physicians to explain how they are reaching the clinical judgments they do (e.g., with the help of standard risk calculators). What is uniquely different about an AI-based system that generates a right to notice and explanation of use and function? Thus, I call for more careful thought and caution in the embrace and implementation of this principle.
Human Alternatives: Ability to Opt-Out, Where Appropriate, and Have Access to Humans
The role of the human in this principle is that they be available to “quickly consider and remedy problems you encounter” with automated systems. This principle also suggests that people have a right to a human over an AI system if they prefer, so long as human access is reasonable given the context. This principle also nods to human governance of AI systems.
Presumably, this principle aims to achieve the idea of keeping a “human in the loop”—an idea embraced in AI technology and development. Human involvement in and governance of AI technologies is important for ethics of use, but we must not lose sight of human biases and the existence of algorithm aversion (anti-AI bias). Patients might be quick to request a human alternative, but for bad reasons, and when the human alternative might be worse. Consider an AI chat bot psychiatrist vs. a human psychiatrist where a patient opts out and requests a human due to algorithm aversion bias, but the human is less accurate at diagnosis, and prescribes medicine or conducts therapy in a less evidence-based way than the bot would. In light of this, I like how this principle is formed (as an opt-out) where the default might be an AI-system if it is safe and effective. Another caution with this principle concerns involving a human to “consider and remedy” problems with automated systems—given humans’ overconfidence biases and anti-AI biases, humans such as physicians might be too quick to go against or counter the judgments of the AI system but be wrong. For example, an AI based morality calculator may predict that a patient has a 3 month survival chance of only 20%, but the physician dismisses the judgment for a much higher survival chance but is wrong—now the patient has exercised their “human alternative” right, but this right has made them worse off, and they haven’t exercised it very autonomously because their preference was driven by a slew of biases rather than considered choice. Or, physicians may deploy or fail to deploy the results of the AI in biased or heterogenous ways, e.g. over-riding for white patients but using AI for black patients.
In sum, the blueprint for an AI Bill of Rights is an important moral and political move to anticipate the future of AI technologies in our lives while mitigating potential negative impacts and protecting human rights. Bioethicists can contribute significantly to considering these proposed rights in an array of contexts. Both normative and empirical work will be needed to understand how these rights can and should be integrated into AI policy and practice.
Works Cited
Blumenthal-Barby, Jennifer, Benjamin Lang, Natalie Dorfman, Holland Kaplan, William B. Hooper, and Kristin Kostick-Quenet. 2022. “Research on the Clinical Translation of Health Care Machine Learning: Ethicists Experiences on Lessons Learned.” The American Journal of Bioethics 22 (5): 1–3. https://doi.org/10.1080/15265161.2022.2059199.
Kostick-Quenet, Kristin M., I. Glenn Cohen, Sara Gerke, Bernard Lo, James Antaki, Faezah Movahedi, Hasna Njah, Lauren Schoen, Jerry E. Estep, and J.S. Blumenthal-Barby. 2022. “Mitigating Racial Bias in Machine Learning.” Journal of Law, Medicine & Ethics 50 (1): 92–100. https://doi.org/10.1017/jme.2022.13.
Office of Science and Technology Policy. 2022. “Blueprint for an AI Bill of Rights: Making Automated Systems Work for The American People.” Https://Www.Whitehouse.Gov. Accessed October 4, 2022. https://www.whitehouse.gov/ostp/ai-bill-of-rights/.
Jennifer Blumenthal-Barby, PhD, MA (@BlumenthalBarby) is a Professor of Medical Ethics at Baylor College of Medicine.