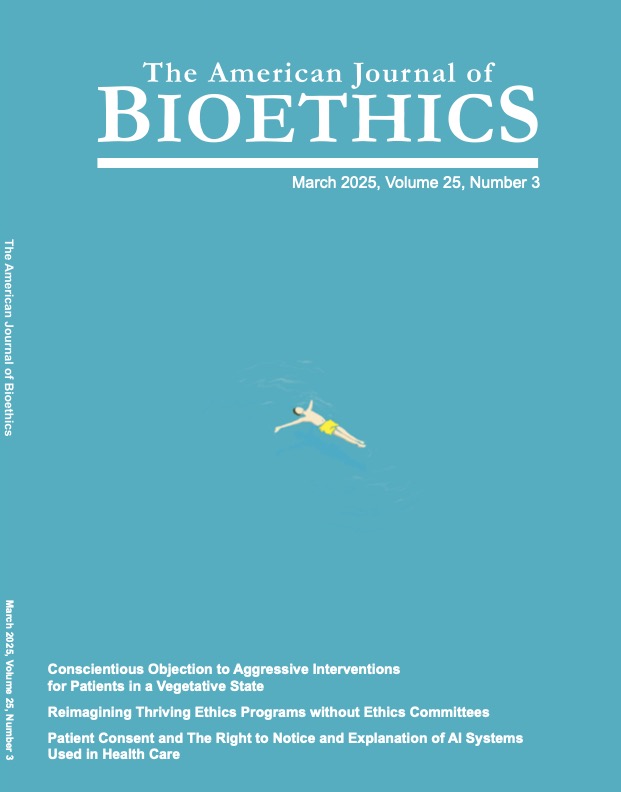
This editorial appears in the March 2025 issue of the American Journal of Bioethics.
As Hurley and colleagues note in this issue of the Journal, transparency in healthcare supports informed patient decision making, promotes trust in healthcare professionals, and encourages patients to learn more about their care. Those who reject transparency do so at great risk, as secrecy in healthcare may be interpreted as disrespectful, arrogant, paternalistic, or self-interested. As such, calls to ensure transparency about the use of artificial intelligence in healthcare are unsurprising and well-supported by familiar principles of biomedical ethics.
Recent guidance from the World Health Organization, the White House’s AI Bill of Rights, and the American Medical Association encourage greater transparency about the use of artificial intelligence in healthcare. These guidelines recommend that patients be informed about when and how AI is used in their care—in the language of the AI Bill of Rights, patients have “the right to notice and explanation”. While transparency is undeniably important in healthcare, achieving it is much more difficult than acknowledged in these calls. Here, we highlight several challenges in pursuing transparency related to the use of AI in healthcare.
Several Features of Healthcare AI Limit Its Potential Transparency
Many forms of healthcare AI are dynamic, with algorithmic adjustments occurring frequently in light of adjustments to training datasets, model weights, and post-deployment tuning in response to real-world performance. Additionally, considerations of security against malicious actors and the protection of private health data and intellectual property may support restrictions on access to detailed characterizations of the inner workings of healthcare AI models. Other applications of AI in healthcare may pose more general challenges related to the scrutability of these systems, not only to their users but to technical AI experts who themselves may be incapable of fully understanding why certain AI systems produce the outputs they do. Full commitment to AI transparency in these cases would clearly not be straightforward, given these limitations.
The novelty of AI applications in healthcare also means that many healthcare professionals have limited familiarity with these tools and how they work. This puts AI in contrast with many other technologies in healthcare, particularly diagnostic technologies, where providers are expected to learn about a tool’s functionality prior to its use.
It is also important to note that the ethical rationale for transparency is unlikely to be achieved by disclosures solely regarding the inner working of AI models. Patients may be interested in how these models were developed, whether their personal health data is being shared with AI developers, and what options they may have if they disagree with a recommendation that has been made by an AI tool. Even details of AI’s implementation may be relevant to patients. The same diagnostic model could independently generate a diagnosis from a patient’s scans, or could run in the background, reading what the provider enters into the chart and only highlighting discordance with its recommendation. Though in these cases the diagnostic model itself is identical, the manner of interaction may impact patients’ comfort with the AI tool. The technology’s deployment thus adds complexity to the pursuit of transparency, potentially increasing the amount of information required for full transparency.
The Diversity of AI Applications in Healthcare Complicates Disclosure Strategies
Returning to the article by Hurley and colleagues, the authors consider several applications of AI in healthcare. The examples they discuss share many similarities. As applications of AI expand, there will be many other use scenarios in healthcare, several of which may require alternative strategies for promoting transparency.
For example, AI tools might play a role in streamlining administrative systems, such as scheduling patient appointments, sending reminders through patient portals, or matching patients to clinical trial opportunities. Other AI tools might be used to improve the operation of healthcare facilities: evaluating data to procure medical supplies, using machine vision to organize hospital parking lots, or analyzing data in support of quality improvement. AI tools might be used to monitor healthcare workers, such as to avoid burnout or to ensure hygiene. AI could also be deployed to promote patient safety, such as tracking the location of tools in a surgical setting to prevent accidental instrument retention. AI might also be deployed in support of medical devices, monitoring those devices and making real-time adjustments to optimize their performance.
It is unclear how healthcare systems might promote transparency about the use of AI in these and other contexts. For example, consider a hospital that uses AI in selecting a specific supplier from which to purchase hand sanitizer. Should each bottle come with a label informing users of the involvement of AI in that decision? Would that label need to include additional details about the AI models’ inner workings? Our moral intuitions are that those types of disclosures would be unnecessary, and if disclosure were required in every instance where AI is deployed in healthcare, these notices and explanations would quickly become overwhelming and potentially distracting to patients and healthcare professionals.
At the very least, there is no clear consensus about when it may be necessary to disclose the use of healthcare AI. While transparency may be a laudable goal, in the future it may be the case that uses of AI are much more common and diverse than imagined by Hurley and colleagues, complicating efforts to promote transparency.