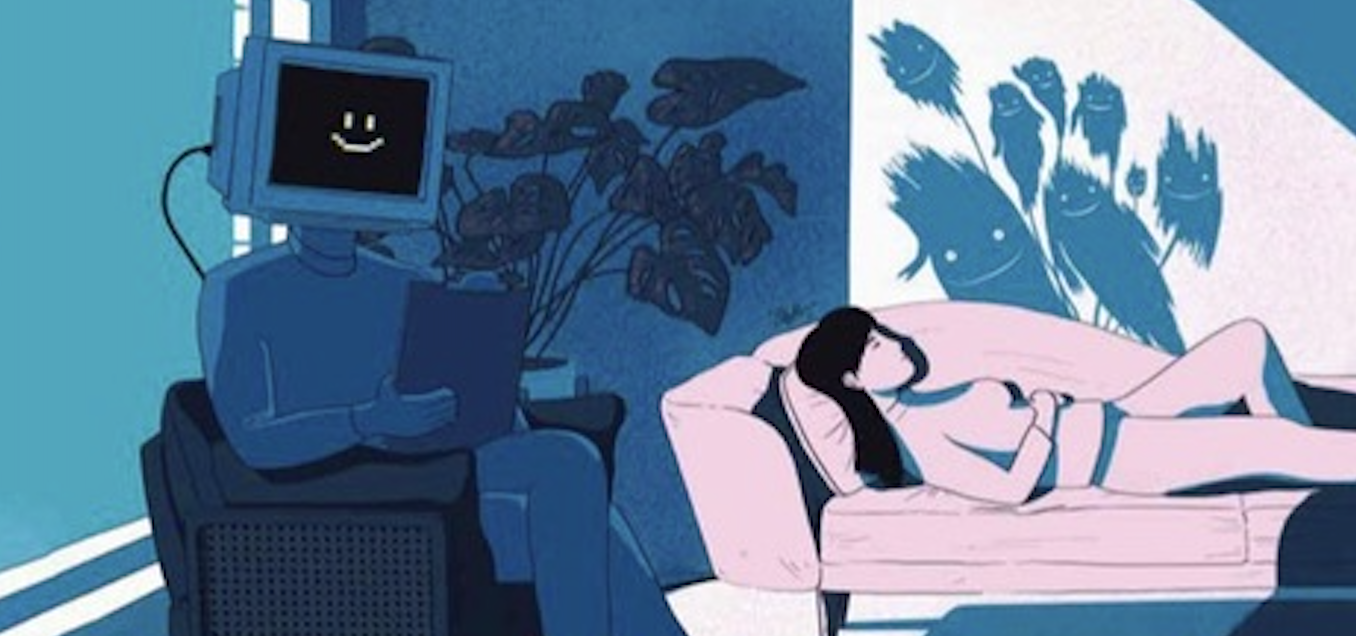
The following editorial can be found in the May 2023 issue of the American Journal of Bioethics.
Good listening is an essential element in the provision of quality healthcare. Good listening also supports accurate diagnosis, patient adherence to medical recommendations, and strong physician-patient partnerships. Patients expect that their healthcare providers will listen to them and often feel disrespected when this is not the case, which may put them at risk of alienation from healthcare providers and medical institutions.
Many emerging artificial intelligence (AI) and digital health tools fail to consider the nuanced communication practices that support good listening, including the various normative elements that undergird quality patient care. In this issue of the journal, Sedlakova and Traschsel consider how the use of a conversational artificial intelligence (CAI) tool raises complex ethical issues related to its use in psychotherapy. The depth of their analysis is noteworthy, as they call attention to the key role that human agency plays in healthcare communication and rebut claims that nonhuman machines will someday be able to approximate therapeutic interactions with human agents. Their analysis supports the idea that inherent limitations of CAI technologies could undermine the authenticity of psychotherapeutic conversations that rely on these tools.
Sedlakova and Traschsel’s analysis also sheds light on what is, at the moment, an important gap in bioethics scholarship related to healthcare AI. As AI and digital health tools are increasingly integrated into patient care, the activities of healthcare teams will likely be augmented by digital tools that do not engage patients in the same manner as human agents. The case of CAI highlights a circumstance in which a machine might process a patient’s verbal or written communication with the goal of offering psychotherapeutic benefit. Adjacent to such applications of CAI, however, are a broader class of AI-enabled tools that can analyze patient communication with a greater range of purposes, namely machine-listening tools.
Not all applications of machine listening seek to replace humans or provide empathetic support for patients. For example, some machine-listening tools do not listen to the content of a patient’s spoken words but focus instead on structural and acoustic components of a patient’s speech. While this is still an emerging area of clinical research, in the future, machine-listening technologies may be able to identify specific vocal biomarkers that support clinical diagnosis. These and other uses of machine-listening technologies are not designed to replace a physician’s judgment, but instead aim to augment clinical decision-making with new types of data.
Machine-listening technologies may be developed in support of various clinical activities, leveraging voice as a key input in making clinical decisions. For instance, patient speech can be analyzed to aid assessments of depression severity or to support the diagnosis of psychiatric conditions. Similarly, voice recordings analyzed using machine learning have been shown to be helpful in the diagnosis of multiple sclerosis and its progression. Beyond clinical prediction and diagnostic capabilities, machine-listening technologies also offer the potential to alleviate administrative burden by automating some aspects of clinical documentation. While these and other forms of machine listening do not challenge the agency of human clinicians in the manner examined by Sedlakova and Traschsel, they nonetheless raise important ethical considerations.
For example, the use of machine-listening tools in clinical settings may impact physician-patient communication by calling attention to the documentation of healthcare discussions. Additionally, machine-listening tools may alter clinical recommendations or create dissonance for physicians who feel that an AI-supported medical recommendation is not consistent with their clinical judgment. Machine-listening tools may also fuel patient worries that their care is being guided not by an empathetic physician but by machine-driven algorithms that might prioritize efficiency over empathy. Cross-cultural issues and concern for vulnerable populations are also important to consider, as these technologies operate within a historical continuum of bias and existing health disparities. Of course, the use of machine-listening technologies holds much promise as well. There is great potential in the ability of these technologies to analyze human voices and patterns of communication beyond the capabilities of human practitioners. Operating against a backdrop of existing norms in health communication, however, due consideration should be given to the ethical and clinical impact of these tools. To date, unfortunately, the ethical dimensions of machine listening have not received much attention. In addition to this lack of a robust normative literature on machine-listening technologies, there has been very little empirical bioethics research done to examine patient and clinician perspectives on these emerging tools, despite prior studies showing that patients have significant concerns about the use of AI tools in their care.
We encourage bioethics scholars to focus more attention on developing an “ethical framework” for assessing machine-listening technologies that seek to improve or extend a physician’s capacity as a good listener. This ethical framework should include, at a minimum, traditional concerns about patient privacy, transparency in the use of machine-listening tools, and appropriate regulation of healthcare AI. The framework should also include considerations related to respectful listening, a topic that is rarely framed as a bioethical concern despite its importance for establishing trusting relationships between patients and healthcare professionals. Lastly, an ethical framework for evaluating machine-listening tools should consider potential dignitary harms associated with the use of these tools in private healthcare settings in which there is a presumption of patient privacy.
Despite significant private investments in machine-listening technologies, ethical guidance on the development and use of these tools in healthcare is extremely limited. It is critical that bioethics scholars address this gap through normative and empirical studies focusing on machine-listening tools.