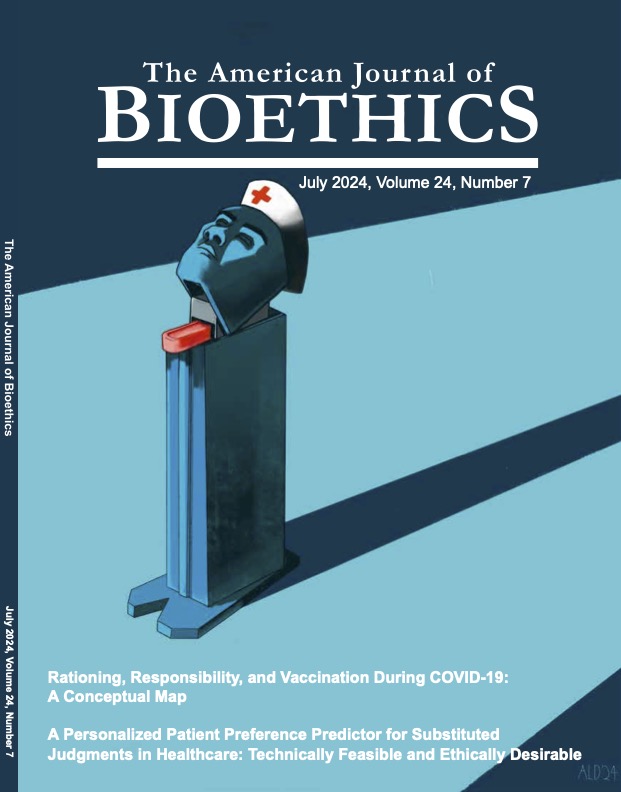
This editorial can be found in the July 2024 issue of the American Journal of Bioethics
ICU with respiratory failure and sepsis. She has been on a ventilator for almost a week, and now has impending kidney failure. Her children, who have been taking turns at the bedside, must soon decide whether to dialyze their mom; and with recovery far from imminent or certain (she experienced brain damage from low blood pressure and oxygenation), they may soon have to decide whether she should get a tracheostomy and a feeding tube. They take in as much medical information as they can, while trying to recall any conversations they’ve had with her that would tell them what she would want them to decide.
In their excellent article in this issue, Earp et al. discuss an algorithm, “The Personalized Patient Preference Predictor (P4),” which they argue could, plausibly, predict the medical treatments an individual would prefer or reject when they cannot express their wishes. They suggest that the development of such an algorithm is technically feasible, given the swift progress in large language models and machine learning technologies. Furthermore, Earp et al. contend that implementing this tool would be ethically desirable.
The technical feasibility of such an algorithm is an empirical matter that we largely set aside. Instead, we concentrate on whether an algorithm like the P4 is as ethically desirable as Earp et al. suggest. From our perspective, a tool like the P4 introduces several significant ethical questions and concerns and downplays some of the complexities surrounding surrogate decision making. Specifically, we raise questions about what data to base predictions on, whether even the best data would lead to accurate predictions, and whether a tool like P4 would make decision making less burdensome for surrogates as opposed to making it more vexing and emotionally complicated. Even more concerning, if P4 performs as well as Earp et al. believe it could, surrogates and clinicians might be morally (or legally) obligated to decide in accordance with the P4’s prediction. After all, it may be considered the ultimate instantiation of substituted judgment, thus thwarting the ability of clinicians and loved ones to pivot toward what they believe will be in the patient’s best interests.
One important issue with an algorithm like the patient preference predictor involves determining the appropriate data to feed into it. Earp et al. suggest that the AI-driven P4 make use of personal data specific to the individual, mitigating concerns that its predictions might be generalized and not truly reflective of the individual involved. Potential data sources they note include emails, blog posts, social media accounts, past medical decisions, recorded or actual conversations, surveys about medical decisions, and even internet browsing and purchase history.
We are genuinely curious what it would mean to verify whether, and how reliably, these sources predict preferences. It will be challenging to train an algorithm to place appropriate weight on social media likes and repostings. In fact, social media algorithms have been shown to shape preferences, sometimes by skewing the information people are exposed to (“click worthy” material) and, too often, by disseminating misleading or blatantly false content. We believe that some of these data sources might be more relevant and appropriate than others, with certain sources leading to inaccurate assumptions about a person’s medical treatment preferences, and more broadly, their genuine desires, beliefs, and preferences.
There are several additional problems. First, these data (especially the most relevant types, such as surveys about medical decisions) will be sparse for most individuals, diminishing the accuracy of P4’s predictions. Second, and more importantly, even when the data are not sparse, relying on the data overlooks insights from decision psychology, which highlight the inaccuracy and instability of expressed preferences. For example, affective forecasting errors cast doubt on patients’ ability to accurately anticipate how they will feel, the extent to which they will adapt, and what they will want in future health states. A person’s social media posts might consistently suggest that they would rather be dead than live with a significant spinal cord injury, but many people who experience such disabilities discover that their quality of life exceeds what they previously anticipated. We expect that a “successful” algorithm would simply mirror these mispredictions.
Third, we are not convinced that use of AI will reduce the emotional burden on families facing difficult surrogate decisions. Suppose Ms. P’s family does not believe she will benefit from dialysis, but that the algorithm suggests employing that treatment. This would likely be quite stressful, with the family struggling to see whether and how Facebook posts from three years ago apply to this unforeseen circumstance. They might have even witnessed subsequent declines in her quality of life, prior to the current hospitalization, that are not reflected in those posts, especially given their mother’s tendency to project a happy image to her friends. They are concerned that their mother is not benefiting from current treatment and her situation and state is not one that would align with her values, goals, and identity as they understood them. What then should be done? Which judgment do we give the most weight and for what moral reasons? Her family’s assessment of her interests and who she was as a person, or a data-generated prediction about her preferences in the situation at hand?
Earp et al. would likely respond that they intend for the P4 to be an adjunct in the process of deciding for incapacitated individuals and for its use to be voluntary. Our concern is that because courts have prioritized substituted judgment (when available) over best interest standards of surrogate decision making, a clinical team might feel bound to treat a tool like P4 as determinative. Moreover, there are psychological reasons that clinicians and family members might treat a tool like P4 to be determinative despite its problems. The P4 purports to deliver a quantitative and direct “answer” to family members and clinical teams struggling with a morally and emotionally complex choice—an attractive prospect. In our view, however, we should be wary of overconfidence in the ability of AI to solve the problems and complexities associated with deciding for incapacitated patients.
Funding
This editorial arose from discussions in the Greenwall Faculty Scholars Philosophical Bioethics Seminar Series, funded by The Greenwall Foundation.