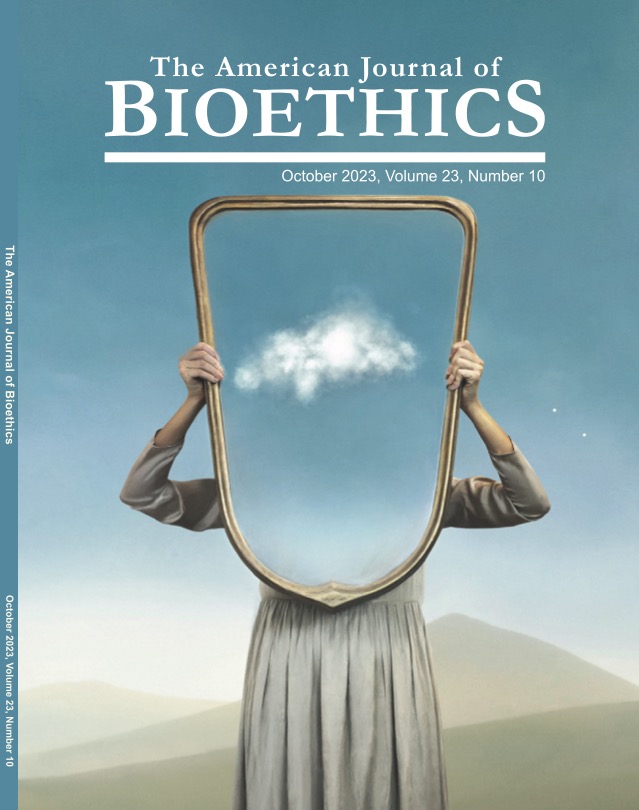
See the following editorial in the November 2023 issue of The American Journal of Bioethics
Recent advancements in large language models (LLMs) have ushered in a transformative phase in artificial intelligence (AI). Unlike conventional AI, LLMs excel in facilitating fluid human–computer dialogues. LLMs in chatbots and ChatGPT have proven capable of mimicking human-like interactions—meeting a demand for various services. These services span from answering electronic health inquiries to acting as mental health support chatbots. The potential for LLMs to transform how we perceive, write, communicate, and utilize AI is profound, emphasizing the importance of understanding the impact of LLMs on human communication.
The use of large language models (LLMs) as a communication tool can have profound social consequences, reshaping human interactions and trust dynamics. A study led by Hohenstein et al. (2023) showed that the efficacy of AI-driven conversations hinges on whether participants knew they were interacting with an algorithm. They found that conversations infused with AI suggestions fostered faster communication and a positive emotional tone. However, when participants thought their counterparts relied on AI, they perceived them as less amiable and cooperative. Another study by Nov, Singh, and Mann, reported similar findings. They found that while patients could not readily distinguish human versus AI-generated responses, they were less likely to trust AI-generated responses for treatment decisions compared to administrative tasks. This finding warrants careful consideration of the use cases where LLMs can be poised to support clinical teams to provide patient care without eroding patient trust when knowing they are interacting with an AI system. Together, these findings point to the fact that despite identical semantic content, attending to the context and human preferences regarding AI-mediated interactions will be important.
Much of the discussion of the capability of AI has operated on the assumption that the actual outputs (the utterances or text) generated by the models are what matters. After all, the goal of the Turing Test (originally called the imitation test) is to see whether someone can tell accurately whether they are communicating with an AI or a human in a decontextualized interaction. And worries that the outputs from LLM’s will be inaccurate or contain false references or claims have been at the forefront of concerns about the technology.
But this perspective misses something important about the nature of language. Language is a tool that people use. People do things with words. The same utterance can mean very different things to different individuals in different contexts. Saying “Do you know what time it is?” can be a request for someone to tell you the time. It can be a complaint about running late when made while tapping your watch, trying to get your partner to finish getting dressed. It can be a literal question as part of a diagnostic evaluation when uttered to someone whose cognition is being tested. Focusing on just the outputs misses important aspects of language. What do we take from the data cited in the preceding paragraphs when thinking about AI-mediated communication between physicians and patients?
First, it may be that accurately conveying information will nonetheless be taken to be a very different type of speech act by patients. The mere fact that an LLM is involved may convey (incorrectly) that the text being sent isn’t important, or that their physician doesn’t have time for them, or that their physician isn’t competent. The impact on the readers or listeners has not yet been adequately studied, but the findings just described suggest that it may matter a great deal. Patients often try to figure out what physicians are intending to convey by the word choices physicians make. But when confronted with communication from an LLM, it is not clear how the words will be taken when there is no intention (because there is no agent).
This creates an ethical dilemma. To what extent should the uses of LLMs in communication with patients be transparent to them? If it turns out that the actual impact of the communicative act is improved through use of AI only as long as the patient is unaware of the origins of the communication, does this justify misleading them? And what would be the impact of discovering that the physician you have been emailing your complaints to, and getting empathetic and appropriate advice from, is really a bot?
It also remains to be seen what the implications are of coping with some of these issues through anthropomorphizing of bots. Attribution of human characteristics to ChatGPT are commonplace, even among experts and engineers. Talk of “hallucinations” and other psychological attributions may have significant epistemological and ethical consequences. ChatGPT does not “think” or “understand” anything. It is a model that predicts what string of words is likely to fit a given query. That is why when you ask “What is the name of Paul’s grandfather’s only grandson?” you will be told that it does not have enough information about Paul to answer the question. As Salles, Evers, and Farisco argue, the anthropomorphic language being used by both developers and users may mask differences between bot and human functioning. ChatGPT does not “hallucinate” and the fictitious references it provides are not a misfunction–-they are a limitation of the correct functioning of the model. Changes in the model or in the training data may lead to performance that more closely matches expectations, but the built-in limitations as a function of the differences in human and bot functioning are sui generis.
We are still in the early days of understanding AI-mediated communication, particularly in clinical contexts. Studies of the pragmatics of communication will be needed to fully address the potential and the pitfalls of these new computer-mediated interactions.
Disclaimer
Any opinions, findings, and conclusions or recommendations expressed in this material are those of the author(s) and do not necessarily reflect the views of the National Science Foundation.
Alaa Youssef, Samantha Stein, Justin Clapp, and David Magnus