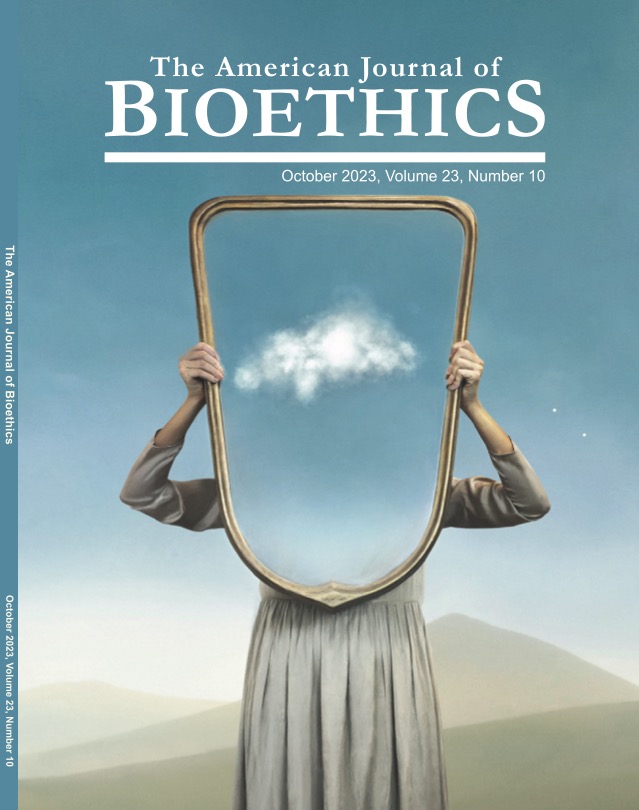
See the following editorial in the November 2023 issue of The American Journal of Bioethics
Introduction
Generative artificial intelligence (GenAI) promises to revolutionize data-driven fields. Building on decades of large language modeling (LLM), GenAI can collect, harmonize, and interpret large amounts of data from diverse inputs including the internet, databases, and electronic medical records (EMRs). It can brainstorm, generate text, and design. Based on uptake, it has been the single most successful product in the western hemisphere.
Integration into healthcare has been swift. Epic, the EMR platform provider which supports the most acute care hospitals in the United States, is piloting the generative pre-trained transformer (GPT-4) GenAI model at UC San Diego Health; UW Health in Madison, Wisconsin; and Stanford Health Care—and is now working on its second wave. And this is just the beginning, GPT-4 is the groundwork for increasingly sophisticated GenAI systems over the next decade.
Because GenAI raises novel challenges—even as compared to previous AI iterations—governments, professional organizations, and even GenAI developers are calling for ethical analysis to inform policy changes. This issue of the American Journal of Bioethics is one such timely response.
In it are three key target articles that will be foundational to the conversation of the impact of GenAI on bioethics moving forward. Glenn Cohen identifies some new, and less new, challenges that GenAI poses for our field from the perspective of integration into clinical medicine. Vasiliki Rahimzadeh and colleagues focus on the impact of LLMs on clinical ethics pedagogy, demonstrating its strengths and weaknesses as compared to their Ethics Work Up approach. And Sebastian Porsdam Mann and colleagues explore the advantages and challenges of training an LLM to brainstorm and write in a way reflective of an existing scholar. Response to these target articles was strong and we are also publishing 28 Open Peer Commentaries (OPCs) that confirm, push back, and build on these ideas. We are proud to be able to jumpstart the conversation of GenAI and bioethics in this way.
Application of GenAI to Health Data Research
But symbiotic with GenAI’s integration into clinical care—and the very generation of clinical data—we also need to understand the impact of GenAI on health data research. GenAI calls into question regulations, policies, and even the underlying philosophy of health data integrity and sharing.
Data integrity and sharing in the United States currently benefit from robust regulation, guidance, and policy to inform expectations and create mechanisms for enforcement. The White House Office of Science and Technology Policy recently announced its goal of ensuring that federally supported research, along with data of sufficient quality to validate and replicate the findings, be made available to the public immediately with publication. Responsive NIH policy recently became effective. Other government institutions have several years to develop their own.
GenAI will challenge these massive and complex federal and institutional health data sharing policies—many of which are only freshly polished. I will join other commentators in building on Cohen’s “less new” versus “new-ish” paradigm by highlighting four things I think are importantly different between AI bioethics scholarship and where GenAI bioethics scholarship will have to go to adequately inform the future of health data research.
Differences Between AI and GenAI for the Ethics of Health Data Research
Natural Language Processing
First, a novel advancement in GenAI as compared to AI is that it has the capacity to produce its own healthcare data. AI generally requires sophisticated coding techniques to program algorithms to classify and organize specific datasets in a targeted way. Use of AI therefore has a natural educational gatekeeper: few have the knowledge base to program and access these advanced techniques. But GenAI is queried by simple language prompts that researchers will be able to produce and incorporate into their work. GenAI assesses massive databases and generates language responses that are based on such queries, but do not exist in the training dataset per se. The responses are based on patterns found in those data, but GenAI interprets them, and generates its own script.
It is of course true, as Rahimzadeh et al. point out, that generating quality responses takes “technical literacy on the part of users,” particularly in prompt design. But that likely only compounds, rather than reduces, the problem of people using GenAI incompetently. In addition, as Lea Goetz and colleagues argue “LLMs are trained to be agreeable,” which Cohen labels “a distinct danger of a good Chatbot…”. GenAI is trained to respond in a way that is comfortable to humans—a way that we like and trust—and perhaps assume better intentions of than of an algorithm that scrapes the internet. As Emily Bender and colleagues put it in 2021: “The ersatz fluency and coherence of [language models] raises several risks, precisely because humans are prepared to interpret strings belonging to languages they speak as meaningful and corresponding to the communicative intent of some individual or group of individuals who have accountability for what is said”. The impact of ChatGPT appearing so…human…in its interactions is an issue that will launch 1,000 academic careers.
Falsification, Fabrication, and Plagiarism
Another novel challenge for GenAI v AI is that GenAI content is sometimes falsified, fabricated, or plagiarized by GenAI itself. GenAI can “hallucinate” (make up) data and sources or plagiarize content (as Nyholm interestingly explores further with respect to authorship). While humans can also make errors, signals researchers use to recognize that human generated data are untrustworthy will be upended. In addition, users may be influenced by GenAI’s perceived “algorithmic neutrality” leading, as Vasiliki et al. argue, to interpretation of GenAI-generated data as “authoritative persuasion”.
GenAI can even make up data intentionally and without notice, for example “jailbreak” (generating data against user guidelines) or disagree with a prompt because it believes its version is better than the one the user asked for. Even GenAI developers urge users to always verify because “it is probably wise not to trust the results”. In addition, in 2021 Bender et al. argued that by not documenting training data as LLMs are built will lead us to “incurring documentation debt i.e. putting ourselves in a situation where the datasets are both undocumented and too large to document post hoc” which is necessary for accountability and transparency. Yet here we are—after a massive technologic race to release the first models to the public—with completely empty pockets.
Biases
Third, while much has been written about how AI can compound biases found in its training datasets, GenAI can amplify biases in ways not yet fully understood. As Skorburg et al. explains “the transformer architectures powering LLMs have far fewer data-specific ‘inductive biases’ than their predecessors. This means that they can easily consume different kinds of data without much domain-specific engineering, rapidly accelerating their multimodal abilities”. In addition, as Matthew Elmore and Victor et al. point out, GenAI is trained on broad interdisciplinary content (rather than, e.g., EMR data from major academic medical centers) and “the actual mosaic of its training data remains opaque even for its developers”. There have been many concerns raised about the biases and homogeny of databases used by AI which can and have resulted in inequitable results for historically marginalized patients. But we don’t even fully understand what is training GenAI to begin with to enable assessment of such risks. Which, to be clear, was an entirely intentional choice.
There are at least some knowns, and some speculations, of at least some GenAI training data. This includes a general learning toward free internet data as opposed to academic firewalled (and peer-reviewed) data. GPT-2 included data from outbound links from Reddit and Wikipedia, whereas most U.S. Reddit users are men between the ages of 18–29 and only 8.8–15% Wikipedia authors are female. LLMs have almost exclusively been trained in English. OpenAI also paid “data enrichment professionals” in Kenya under $2 per hour to clear the datasets of the “darkest recesses of the internet”. The employees scrubbed datasets of targeted keywords that might have been used derogatorily…or might have been part of reclaimed discourse of marginalized populations. And, as Elise Li Zheng & Sandra Soo-Jin Lee eloquently explain, “When categories of experience fail to register because groups do not have epistemic authority, there is the risk that these elisions will have significant downstream impact”.
Moral Accountability for Data Integrity
A last area is how GenAI also challenges our moral foundations for, and taxonomy of data integrity. The moral standard by which federal funding agencies and universities judge such violations is by defining the appropriate mens rea, or intentionality to commit, such that the violation must be intentional, knowing, or reckless. Porsdam Mann et al. focus on the positive side of this balance from the perspective of “credit-blame asymmetry” i.e. that individuals will be held blameworthy for LLM-generated content which is “bad” yet will not gain much credit for the generation of content which is “good,” but more work needs to be done to define the how and why of that blameworthiness. The NIH Deputy Director of Extramural Research has warned that investigators use GenAI for their grants “at their own risk”. But what should the standards be?
Of course, our current standards for holding authors responsible for the integrity of their data is already flawed. Look no further than the infamous Lancet and New England Journal retractions by the same authorship team regarding their “research” on COVID-19 and hydroxycholoroquine. The World Health Organization had already paused global clinical trials involving thousands of COVID patients before it was revealed that the lead authors had neither accessed nor reviewed the data upon which their findings were based—and the results were entirely fabricated. The system certainly failed to ensure that all authors reviewed and verified all data before publication, and the publication had negative impact before being retracted. But it was effective at least in catching those errors post-publication and holding authors accountable. We will need to ensure that the moral accountability of the integrity of GenAI generated data is specifically structured and enforced moving forward.
Conclusion
I share Cohen’s excitement for the impact of GenAI on research and am particularly looking forward to tools promising redirecting researcher efforts to the things that we (at least think) need the human mind to produce. But I am both dismayed as a lawyer for how GenAI might unravel some of the excellent federal and institutional work already accomplished in data research policy, yet intrigued as a bioethicist for the novel intellectual challenges this new technology poses.
Throughout the debate, however, it is critical not to lose focus on the two most important generative-AI-generated challenges for health data research:
1.
1. Data sharing and integrity is the backbone of the medical and scientific research enterprise. We have struggled to ensure and enforce standards for validity, reproducibility, transparency, and replicability as it is. The bioethics community needs to be able to quickly identify new challenges posed by GenAI, elucidate the ethical tensions they create, and suggest and support responsive policy.
2.
2. Achieving health equity is the most important challenge facing bioethics today. We were far from close to begin with, and only starting to measure and explore the impact of known data biases on health outcomes. The (fully intentional) “document debt” that the developers of GenAI accrued—and end users are expected to carry—is overwhelming. We will need the field of bioethics to assess what advantages of GenAI are worth improving, how to limit those which are not, and the ethical acumen to know the difference.
We hope that this issue of AJOB, and all the excellent ideas contained herein, is a step in that direction.
Kayte Spector-Bagdady, JD, MBE is an Assistant Professor at University o Michigan Medical School.